Kaiser Permanente study finds artificial intelligence algorithms work better than commonly used breast cancer risk prediction tool
Artificial intelligence algorithms trained to read mammograms were a better predictor of a woman’s future breast cancer risk than a commonly used breast cancer risk assessment tool, a new Kaiser Permanente study found.
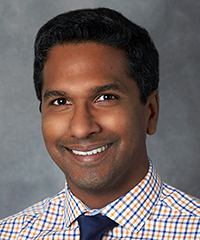
The research, published June 6 in Radiology, suggests the risk-related imaging biomarkers seen on mammograms — that computers can identify and use to generate a risk score — may help doctors provide women with more personalized breast cancer screening recommendations.
“We need to have more accurate and efficient ways to assess a woman’s future breast cancer risk,” said study lead author Vignesh Arasu, MD, PhD, a research scientist at the Kaiser Permanente Division of Research and radiologist specializing in breast imaging with The Permanente Medical Group. “Our study shows that in mammograms that appear negative to a radiologist — meaning there is no sign of a tumor — there is information that computers can see that can aid in assessing a woman’s risk of being diagnosed with breast cancer in the next 5 years.”
The retrospective study included 324,000 women who had a mammogram that found no sign of breast cancer in 2016 at Kaiser Permanente Northern California. None of the women had previously had breast cancer or had been diagnosed with a genetic mutation that increases breast cancer risk. About 24% of the women were younger than 50 when they had their mammogram, and about 87% had no first degree relative with a history of breast cancer.
Over the next 5 years, 4,584 of the women were diagnosed with breast cancer. Of these, 83% were diagnosed with invasive breast cancer and 17% were diagnosed with non-invasive ductal carcinoma in situ (DCIS). The study compared these women with 13,435 of the 324,000 women in the original group who did not develop breast cancer.
The researchers had 5 artificial intelligence (AI) algorithms report a 5-year breast cancer risk prediction score from the mammography images made for these women in 2016.
These algorithms can also see imaging biomarkers on mammograms that tell us there are changes happening in the breast tissue that can be used to predict risk.”
— Vignesh Arasu, MD, PhD
The researchers also used the Breast Cancer Surveillance Consortium (BCSC) clinical risk model to assess each woman’s risk of developing breast cancer over the next 5 years. The model predicts risk using age, race or ethnicity, first-degree family history of breast cancer, number of prior benign breast biopsies, and mammographic breast density. Each woman’s score was calculated using clinical data in her electronic health record at the time of her mammogram in 2016.
The study showed that the women with the highest BCSC risk scores — the top 90th percentile — accounted for 21.1% of all cancers over the next 5 years. In comparison, the women with the highest AI risk scores accounted for 24% to 28% of all cancers.
“Right now, most AI algorithms trained to read mammograms are used to flag a visible cancer to assist radiologists,” said Arasu. “But these algorithms can also see imaging biomarkers on mammograms — in addition to breast density — that tell us there are changes happening in the breast tissue that can be used to predict risk.”
The researchers also used a statistical method called area under the curve (AUC) to compare how well each risk model predicted which women would be diagnosed with breast cancer within the next 5 years. An AUC result can range from 0.0 (no correct predictions) to 1.0 (all predictions were correct). The BCSC model had an AUC of 0.61. In comparison, the AI models had a risk prediction result that ranged from 0.63 to 0.67.
When the AI and BSCS risk predictions were combined, the AUC ranged from 0.66 to 0.68, which was higher than all individual AI algorithms. The combined model did even better at predicting women who had an interval breast cancer diagnosis within a year of their mammogram, with an AUC as high as 0.73. An interval breast cancer is a tumor that is diagnosed — typically because a woman finds a lump in her breast — during the time between a screening mammogram that appears normal and her next scheduled screening mammogram. Interval cancers are typically fast-growing cancers that are more likely to spread to other parts of the body.
“The better predictive ability for interval cancers tells us that AI is identifying aggressive breast cancers previously missed by routine screening,” said Arasu.
The researchers say their findings suggest that AI used alone or combined with current risk prediction models provides a new avenue for future risk prediction.
“Currently, clinical radiologists are mainly using AI with mammography to identify a breast tumor,” said Arasu. “But the findings from our study support using AI to produce a predictive risk score that can tell us a woman’s risk of being diagnosed with breast cancer over the next 5 years. Using that information along with other known risk factors could help us move away from one-size-fits-all screening recommendations and developing personalized breast cancer screening recommendations for women based on their individual risk factors.”
The study was funded by The Permanente Medical Group’s Delivery Science and Applied Research Program and the National Cancer Institute.
Co-authors include Laurel A. Habel, PhD, Ninah S. Achacoso, MS, Lawrence H. Kushi, ScD, Vincent X. Liu, MD, Caitlin M. Lydon, MPH, and Catherine Lee, PhD, of the Division of Research; Diana S.M. Buist, PhD, of the Kaiser Permanente Washington Health Research Institute; Jason B. Cord, MD, and Donald A. Lewis, MS, of the Southern California Permanente Medical Group; Laura J. Esserman, MD, M. Maria Glymour, ScD, Nola M. Hylton, PhD, and John Kornak, PhD, of the University of California, San Francisco; Diana L. Miglioretti, PhD, of the University of California, Davis; Daniel A. Navarro, MD, of The Permanente Medical Group; Albert Pu, MS, of Kaiser Foundation Hospitals; Li Shen, PhD, and Weiva Sieh, MD, of the Icahn School of Medicine at Mount Sinai; and Hyo-Chun Yoon, MD, PhD, of the Hawaii Permanente Medical Group.
# # #
About the Kaiser Permanente Division of Research
The Kaiser Permanente Division of Research conducts, publishes and disseminates epidemiologic and health services research to improve the health and medical care of Kaiser Permanente members and society at large. It seeks to understand the determinants of illness and well-being, and to improve the quality and cost-effectiveness of health care. Currently, DOR’s 600-plus staff is working on more than 450 epidemiological and health services research projects. For more information, visit divisionofresearch.kaiserpermanente.org or follow us @KPDOR.
This Post Has 0 Comments