Large Kaiser Permanente study examines rates of mistriage
A Kaiser Permanente study looking at the application of the Emergency Severity Index (ESI) — a method commonly used to triage emergency department (ED) patients — found some imprecision, suggesting there may be an opportunity for the ESI to be updated.
The study, which included more than 5 million patient visits at Kaiser Permanente Northern California emergency departments, found the method underestimated the severity of patient conditions 3% of the time, but overestimated severity about 25% of the time. The results were published in JAMA Network Open.
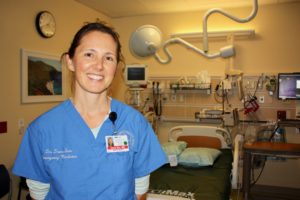
The ESI was developed in the 1990s by emergency physicians — with additional input by emergency nurses — and uses vital signs, patient observation, and questions regarding patient symptoms. Patients are then assigned to one of 5 severity levels that emergency departments use to prioritize patients before they are evaluated by a care team. Two-thirds of U.S. hospitals use this long-standing triage method.
“The ESI was developed before predictive modeling,” said lead author Dana Sax, MD, an adjunct investigator with the Kaiser Permanente Division of Research and emergency medicine physician with The Permanente Medical Group. “The subjective nature of the algorithm does not account for past medical history from the electronic health record to help assign the score. While it’s better to err on the side of overestimation of patient acuity, over-triage has its disadvantages including slowing down patient care in the emergency department and risking unnecessary use of hospital resources.”
This study evaluated the ESI’s effectiveness in appropriately moving patients through the emergency department and did not examine negative impacts on patient care. Sax said the research team, affiliated with the Kaiser Permanente CREST research network, will be looking more deeply into effects on patients in future research.
Past studies have evaluated the ESI’s performance, but they relied on manual review of a relatively small number of patient charts, the authors said. In this study, 5 emergency medicine physicians and 3 emergency nurses developed a set of objective definitions of under- and over-triage and applied them to millions of patients seen between 2016 and 2020.
For the under-triaged patients, most were assigned mid-level severity when they should have been one level higher. The authors identified several factors that increased patients’ risk of under-triage, including use of certain higher-risk prescriptions, having other chronic illnesses, and having recently been hospitalized in the intensive care unit. These risk factors could be flagged for consideration at triage to more accurately categorize patients for care, the authors said.
Over-triage may occur for various reasons, including triage nurses’ efforts to avoid missing sicker patients, or variation in how doctors practice, making prediction of resource use at triage inherently challenging.
The study found more than 60% of encounters were assigned the mid-level triage category (ESI Level III), and 98% were assigned into the middle 3 of 5 severity categories (ESI Levels II, III, or IV). This suggests poor differentiation of patient acuity using ESI and that “a 5-level system may not really be that useful,” Sax said.
The study also found differences by race and ethnicity, gender, socioeconomic status, and arrival time. Over- and under-triage was more likely among young, Black, Latino, and male patients; those living in poorer neighborhoods; and those arriving at night and on weekends. Although the exact reasons for these observations are unknown, these findings highlight an opportunity to standardize triage practices to improve care and equity, the authors said.
Potential role for machine learning
The researchers noted the inherent difficulty in evaluating a patient and reviewing all relevant clinical information to make a triage determination in only a few minutes. “Emergency nurses need to rapidly decide which patient gets the bed and the resources, often deciding while they look at a sea of other people presenting for care,” said study co-author Tina Vitale, RN, emergency department director at Kaiser Permanente’s San Rafael, Calif., medical center.
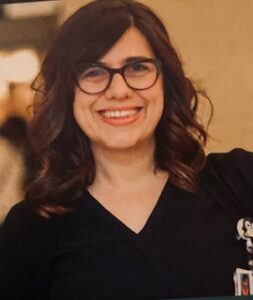
But modern electronic health record systems can be designed to present clinical information thoughtfully to cue providers to high-risk situations and assist with decision-making, Sax noted. While this is already happening for other conditions such as sepsis, the ESI algorithm does not currently make use of data available in the electronic health record to assist with decision-making, offering an opportunity for improving the ESI, she said.
Aiming to improve accuracy and equity of triage practices, the research team is also developing new predictive models for emergency department triage that use machine learning, a sophisticated data analysis method that can compute large amounts of data to calculate predicted risks. One model under development predicts higher-acuity patients at triage who are likely to need hospitalization, and another predicts low-acuity patients who could potentially be seen in an urgent care or a fast-track model emergency department. The team is seeking federal funding to develop and implement the models.
“On average, about 11% of ED patients end up admitted to the hospital, and about 40% of ED patients are low-acuity and could be served in fast-track spaces,” Sax said. “If we could identify those patients early, it could decrease crowding in the main ED.”
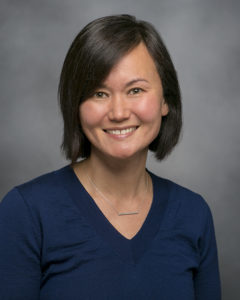
The study team’s plans for large-scale evaluation of a new and improved severity score could result in valuable insights for emergency medicine and nursing, even outside of the Kaiser Permanente system, said senior author Mary Reed, DrPH, research scientist with the Division of Research. “This kind of large data-driven predictive analysis is both novel and important,” Reed said. “We are fortunate to have both the data and the infrastructure at Kaiser Permanente to address accuracy of triage, and to improve equity.”
The study was funded by the Kaiser Permanente Lokahi Risk Reduction program.
Additional co-authors were Margaret Warton, MPH, of the Division of Research; Dustin G. Mark, MD, David R. Vinson, MD, Dustin Ballard, MD, Mamata V. Kene, MD, of The Permanente Medical Group; Katherine R. McGaughey, RN, and Aaron Beardsley, RN, of the Kaiser Permanente Oakland Medical Center; and Jesse M. Pines, MD, MBA, of U.S. Acute Care Solutions.
About the Kaiser Permanente Division of Research
The Kaiser Permanente Division of Research conducts, publishes and disseminates epidemiologic and health services research to improve the health and medical care of Kaiser Permanente members and society at large. It seeks to understand the determinants of illness and well-being, and to improve the quality and cost-effectiveness of health care. Currently, DOR’s 600-plus staff is working on more than 450 epidemiological and health services research projects. For more information, visit divisionofresearch.kaiserpermanente.org or follow us @KPDOR.
This Post Has 0 Comments