Kaiser Permanente research suggests suicide risk calculations could assist care of new patients
A computer model trained on medical records and applied to the past medical history of nearly a million Kaiser Permanente Northern California (KPNC) patients was able to identify many patients at risk for later suicide attempts. The research was published in JAMA Psychiatry.
The study examined whether an existing risk calculation approach would work when considering patients who are newly seeking treatment. The model was accurate even though new patients often lack extensive mental health history in their records, the authors found.
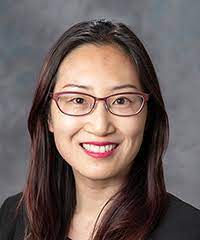
It is important to identify a patient’s risk status early in their treatment, the authors said, because many patients stop mental health treatment after the first or second visit. Clinicians can use model results as one element in their existing, comprehensive assessment.
“Clinical judgment remains the gold standard,” said co-author Honor Hsin, MD, PhD, a psychiatrist with The Permanente Medical Group. “This risk model provides one additional piece of information as clinicians assess and plan treatment with their patients.”
The study used a predictive model that was developed, tested, and validated in 7 health systems — not including KPNC — by the Mental Health Research Network (MHRN). The researchers with Kaiser Permanente’s Division of Research (DOR) carried out the test to apply it to the KPNC population.
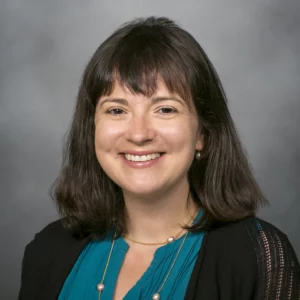
“This model has already been developed and validated, and now the research is focusing on what we can do with this powerful tool,” said senior author Esti Iturralde, PhD, a DOR research scientist. “This study takes us another step forward in understanding how the predictive model would work with a particular group of patients — those seeking mental health care for the first time, rather than those in ongoing care.”
The model was not designed to predict immediate risk of self-harm, but rather the potential for self-harm over the following 90 days. That knowledge could have value for clinicians as they establish relationships with these new patients, Iturralde said.
Analysis of 1.6 million appointments
The analysis applied the MHRN model to 1,623,232 scheduled mental health intake appointments between January 2012 and April 2022. The dataset included 835,616 patients; their intake appointments may have been a first-time mental health visit in general or to a new KPNC clinic, or the first visit after a gap of 2 or more years.
The researchers looked for suicide attempts recorded in the medical record at any point 1 to 90 days after the scheduled appointment.
They found 2,800 suicide attempts, after 0.17% of the total visits. The model showed good overall classification performance, the authors said; the 10% of appointments identified at the highest risk level accounted for nearly half of the appointments followed by a suicide attempt within 90 days.
The model is summarizing the wisdom of many clinicians who have interacted with this patient in the past and is providing another data point for the new clinician to inform their clinical judgment.
—Santiago Papini, PhD
“This study suggests that applying the model at this early point in a patient’s journey can give us useful information,” Hsin said. “This analysis was a necessary step and serves as a foundation for determining if we could use it in the real world.”
In standard practice, clinicians ask patients about suicidal thoughts, but many people may be reluctant to reveal that with a clinician new to them, Iturralde said. “The benefit of a model like this is it pulls from past information about a patient, such as previous medications or past diagnoses,” she said. “It may provide more context about the patient or reveal risk that is not evident through the other tools clinicians use.”
A role for AI in suicide prevention
Predictive models such as this one have the potential to assist clinicians, said lead author Santiago Papini, PhD, assistant professor at the University of Hawaiʻi at Mānoa, who carried out the analysis while a Delivery Science/Informatics fellow with the Division of Research. “We use the term ‘augmented intelligence’ rather than ‘artificial intelligence’ because of the technology’s supporting role,” Papini said.
“The model is summarizing the wisdom of many clinicians who have interacted with this patient in the past and is providing another data point for the new clinician to inform their clinical judgment,” he added. “It’s not replacing their clinical judgment.”
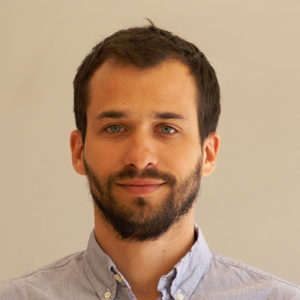
The predictive model was developed using machine learning. This allowed the developers to use large amounts of data for the computer to learn complex patterns and improve the predictive accuracy of the model. The original model was developed using 10 million mental health outpatient visits and 300 predictive types of patient data.
The model’s application in real-world care requires calibrating it to avoid missing at-risk patients while not overwhelming the system by flagging low-risk patients, Papini said. Continuing research will explore how to best incorporate this tool to the workflow of clinicians and examine whether real-world use of the model can benefit patients, he said.
“Since the pandemic began we’ve seen a big increase in patients seeking mental health care, and we’ll need innovations like this to ensure that vulnerable patients are not missed,” Papini said.
The study was supported by The Permanente Medical Group Delivery Science and Applied Research program.
Additional co-authors were Vincent Liu, MD, MS, Patricia Kipnis, PhD, Yin Lu, MD, MPH, and Stacy Sterling, DrPH, MSW, MPH, of the Division of Research; and Kristine Girard, MD, of The Permanente Medical Group.
# # #
About the Kaiser Permanente Division of Research
The Kaiser Permanente Division of Research conducts, publishes and disseminates epidemiologic and health services research to improve the health and medical care of Kaiser Permanente members and society at large. It seeks to understand the determinants of illness and well-being, and to improve the quality and cost-effectiveness of health care. Currently, DOR’s 600-plus staff is working on more than 450 epidemiological and health services research projects. For more information, visit divisionofresearch.kaiserpermanente.org or follow us @KPDOR.
This Post Has 0 Comments