Identifying dozens of subsets within sepsis could lead to more tailored treatment
Sepsis is a potentially lethal and complex condition affecting patients who all have serious infections but may otherwise appear to have little in common. A new machine learning algorithm developed by Kaiser Permanente researchers reveals the complexity of sepsis by sorting patients into recognizable treatment subgroups.
Sepsis kills an estimated 6 million hospitalized patients worldwide each year. Infected patients can rapidly deteriorate as the body overreacts to infection, leading to organ failure. Hospital clinicians have made major strides in identifying sepsis early so they can intervene with timely antibiotic and fluid treatments. But because sepsis patients don’t all look the same, there are limits to this “one size fits all” treatment approach.
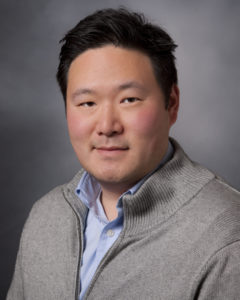
Researchers with the Kaiser Permanente Northern California (KPNC) Division of Research applied an intensive machine learning approach to group sepsis patients by the various diseases and conditions leading to their hospitalization. The results were published July 17 in the Journal of the American Medical Informatics Association (JAMIA).
Senior author Vincent Liu, MD, MSc, a critical care physician and research scientist at the Division of Research, said the results reveal what clinicians are dealing with every day.
“Sepsis patients have incredibly diverse presentations and combinations of health conditions. Instead of simply grouping them under a single ‘sepsis’ umbrella, we now can develop more targeted research and treatment guidelines,” Dr. Liu said.
The research team applied computational algorithms developed outside of health care to the electronic health records of 29,253 adults who had been diagnosed with sepsis in the emergency department and admitted to KPNC hospitals between 2010 and 2013. The analysis looked for hidden patterns in orders, medications, and procedures early in the hospital stay.
By using detailed electronic health data, they grouped sepsis treatment patterns into 42 clinically recognizable treatment topics, such as cellulitis, atypical pneumonia, or mechanical ventilation. The analysis then produced a clinical signature for each patient, representing the different types of treatments patterns patients received.
‘Colorful array of subgroups’
Because of the tremendous diversity within sepsis, the authors found it difficult to pin a single label on many patients; most hospitalizations did not exhibit a single dominant treatment pattern.
“When we used machine learning to examine these patterns closely, it turns out that there are very few sepsis patients that can be simply labeled as having ‘pneumonia’,” Dr. Liu said. “Instead, there are pneumonia patients with cellulitis, those with heart failure, those with diabetes, and any number of other combinations.”
When they plotted these combinations for each patient, they were surprised by how many combinations were represented. “Where we were expecting urinary tract infection or diarrhea,” said Dr. Liu. “We came up with very a colorful array of subgroups. For the first time, this illustration vividly displays just how complex and heterogeneous sepsis patients are.”
Liu sees “sepsis” as an umbrella term, much like “cancer.” And just as cancer research and treatment depends on accurate subtypes, Dr. Liu believes sepsis patients need to be better understood and treated in subgroups.
“You wouldn’t give the same treatment to everyone with cancer even if it’s in the lung, and it’s the same with sepsis,” Dr. Liu said. “In addition to the rich clinical data we have available in electronic health records, we also need to pair that with genetic and biomolecular data.”
Lead author Alison E. Fohner, PhD, assistant professor at the University of Washington, worked on the project as a delivery science fellow in informatics at Kaiser Permanente’s Division of Research, where she remains an adjunct researcher. Fohner adapted machine learning algorithms developed by computer scientists for non-health data to manage the huge amounts of data involved. “We ended up using 2 billion data points but were able to run the entire process in about an hour,” she explained. Future use of their approach using more powerful computing platforms could make groupings available at the bedside.
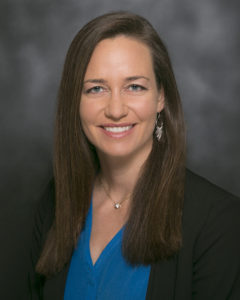
Kaiser Permanente is in a unique position to develop big data projects such as this, Fohner said, because it is an integrated health care delivery system whose extensive medical records system can be plumbed using more specific treatment terms than might be possible examining other data sets containing more generic diagnosis or billing code terminology.
The team’s approach in sepsis could also easily be applied to better understand other medical conditions as well, Fohner said, though the approach is atypical for epidemiology.
“A study like this is very exciting and represents a new approach,” Fohner said. “These methods get us past some of the traditional barriers in epidemiology, where so much of what we study is based on what people have studied already.”
The work was funded by The Permanente Medical Group, the Kaiser Permanente Division of Research Delivery Science Fellowship, and the National Institutes of Health.
Other authors included Gabriel J. Escobar, MD, John D. Greene, MA, Brian L. Lawson, MS, and Patricia Kipnis, PhD, of the Kaiser Permanente Division of Research; and Jonathan H. Chen, MD, PhD, of the Stanford University Division of Biomedical Informatics Research.
This Post Has 0 Comments